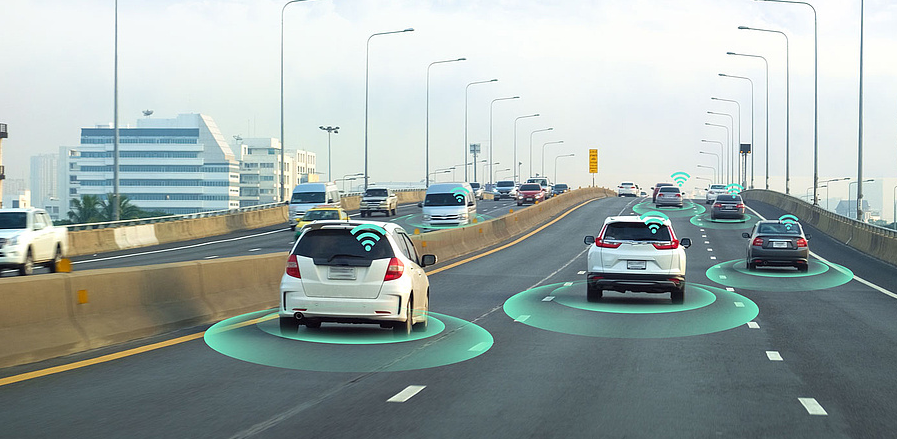
In order for the driver assistance and safety systems in modern cars to sense their environment and operate reliably in any situation, they must rely on sensors such as cameras, lidar, ultrasonic and radar. The latter in particular is an integral component. Radar sensors provide the vehicle with position and velocity information from surrounding objects. However, they have to deal with the numerous damaging and environmental impacts of traffic. Interference from other (radar) equipment and extreme weather conditions create noise that negatively affects the quality of radar measurements.
"The better the denoising of interfering signals, the more reliable the position and velocity of objects can be determined," explains Franz Pernkopf from the Institute of Signal Processing and Speech Communication. Together with partners such as Infineon, his team has developed a neural network-based artificial intelligence system that can mitigate mutual interference in radar signals far beyond the current state of the art. They now want to optimize the model so that it works outside of the learned mode and recognizes objects more reliably.
Resource efficient intelligent signal processing
To this end, the researchers first developed an automatic noise suppression model architecture based on so-called convolutional neural networks (CNNs). "These architectures are modeled on the hierarchy of our visual cortex and have been used successfully for image and signal processing," Pernkopf said. CNNs filter visual information, identify connections and complete images using familiar patterns. Due to their structure, they consume far less memory than other neural networks, but still exceed the available capacity of radar sensors for autonomous driving.
Compress AI models
All aim is to make interference filtering more efficient. To do this, the TU Graz team trained various neural networks with noisy data and desired output values. In experiments, they identified a small and fast model architecture by analyzing the memory space and the number of computational operations required for each denoising process. It is then compressed again to the most efficient model by reducing the bit width. Finally, an AI model with high noise filtering and low energy consumption is realized. Excellent denoising results, with an F1-score (a measure of test accuracy) of 89%, almost equivalent to the object detection rate of an undisturbed radar signal. Consequently, interfering signals are almost completely removed from the measurement signal.
In numbers: The 8-bit wide model achieves the same performance as its 32-bit wide counterpart, but requires only 218KB of memory. This equates to a 75% reduction in storage space, meaning the model far exceeds the current state-of-the-art.
Focus on robustness and interpretability
In the FFG project REPAIR (Robust and ExPlainable AI for Radarsensors), Pernkopf and his team are now working with Infineon to optimize their development over the next three years. Pernkopf said: “We used data (interfering signals) similar to the data we used for training. We now need to improve the model so that it remains valid when the input signal deviates significantly from the learned pattern. This will make the radar sensor robust to environmental disturbances. Rod performance is many times higher. After all, sensors are also faced with different and sometimes unknown situations in reality. Until now, even the smallest change in measurement data was enough to cause the system output to crash so that it could not detect an object or Falsely detected, which would be devastating in autonomous driving use cases.”
The Products You May Be Interested In
![]() |
AIAP-01-272K-T | FIXED IND 2.7MH 90MA 25 OHM TH | 16989 More on Order |
![]() |
AMPMEEC-50.0000T3 | MEMS OSC XO 50.0000MHZ CMOS SMD | 461 More on Order |
![]() |
AMPMGEA-10.0000T | MEMS OSC XO 10.0000MHZ CMOS SMD | 375 More on Order |
![]() |
AMPMDGD-10.0000T | MEMS OSC XO 10.0000MHZ CMOS SMD | 178 More on Order |
![]() |
AMPMDFC-20.0000T | MEMS OSC XO 20.0000MHZ CMOS SMD | 447 More on Order |
![]() |
AMPMDDC-15.3600T | MEMS OSC XO 15.3600MHZ CMOS SMD | 454 More on Order |
![]() |
AMPMEGB-34.0000 | MEMS OSC XO 34.0000MHZ CMOS SMD | 354 More on Order |
![]() |
AMPMGGA-66.0000 | MEMS OSC XO 66.0000MHZ CMOS SMD | 415 More on Order |
![]() |
AMPMAFD-6.1440 | MEMS OSC XO 6.1440MHZ CMOS SMD | 383 More on Order |
![]() |
ASGTX-D-311.040MHZ-2-T | XTAL OSC VCTCXO 311.0400MHZ LVDS | 426 More on Order |
![]() |
AX7MBF1-1120.0000C | XTAL OSC XO 1.1200GHZ CML SMD | 296 More on Order |
![]() |
AX7DBF2-960.0000C | XTAL OSC XO 960.0000MHZ LVDS SMD | 478 More on Order |
![]() |
AX7DBF1-644.5205T | XTAL OSC XO 644.5205MHZ LVDS SMD | 493 More on Order |
![]() |
AX5HBF1-311.0400C | OSC XO 311.04MHZ 2.5V HCSL | 303 More on Order |
![]() |
AX5DBF3-150.0000T | OSC XO 150MHZ 2.5V LVDS | 170 More on Order |
![]() |
ASTMHTE-12.288MHZ-XJ-E-T | MEMS OSC XO 12.2880MHZ LVCMOS | 295 More on Order |
![]() |
ASTMHTE-12.000MHZ-XK-E-T3 | MEMS OSC XO 12.0000MHZ LVCMOS | 282 More on Order |
![]() |
ABM13W-80.0000MHZ-7-B2X-T5 | CRYSTAL 80MHZ 7PF SMD | 267 More on Order |
![]() |
ABM13W-48.0000MHZ-8-J1Z-T5 | CRYSTAL 48MHZ 8PF SMD | 321 More on Order |
![]() |
ABM12W-30.0000MHZ-8-J1Z-T3 | CRYSTAL 30.0000MHZ 8PF SMD | 398 More on Order |
![]() |
ABM8W-19.6608MHZ-8-K1Z-T3 | CRYSTAL 19.6608MHZ 8PF SMD | 285 More on Order |
![]() |
ABM8W-13.5672MHZ-4-B1U-T3 | CRYSTAL 13.5672MHZ 4PF SMD | 323 More on Order |
![]() |
ABLS2-16.000MHZ-B1U-T | CRYSTAL 16.0000MHZ 18PF SMD | 254 More on Order |
![]() |
ABLS7M2-24.576MHZ-D2Y-T | CRYSTAL 24.5760MHZ 18PF SMD | 338 More on Order |